Super-resolution and object detection from drones and satellites
Social distancing AI in the era of COVID-19
– Published 20 November 2020
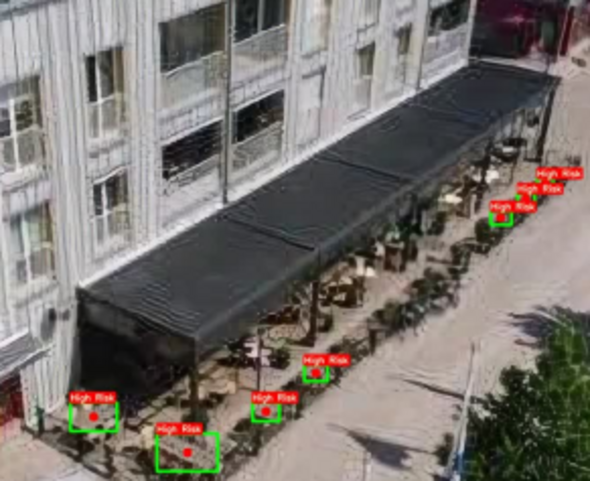
Docent Alexandros Sopasakis and Masters student Dennis Hein at the Centre for Mathematical Sciences, in collaboration with the city of Lund, have been using areal data from satellites and drones to produce machine learning algorithms, which super-resolves the images and subsequently can measure placement of tables in restaurant in outdoor areas. The method can also be implemented for applications within detecting road conditions in traffic or crop condition monitoring in farming.
Super-resolution is a technique that takes a lower resolution image and "paints" in extra details based on expectations dictated by probability rules. The end result being to produce a higher resolution output. Applications range from improving microscopy images to crop monitoring for irrigation, forest fire prevention and even social distancing monitoring from satellites.
Specifically decisions are based on Shannon information theory and differences in measures are computed by the Kullback-Leibler divergence. These tools are included in Generative Adversarial Networks (GANs) which we implemented and calibrated in order to achieve the desired result. Specifically for super-resolution we use ESRGAN which is based on RaGAN. As expected our technique only works in those cases where during learning a number of the images must be annotated in advance. We find that if the contrast between objects of interest and the background is over a threshold, then super-resolution will result in much clearer images. In this particular scenario, using super-resolution to train object detection algorithms represents a feasible solution to the hypothetical distancing scenario this work tries to resolve.